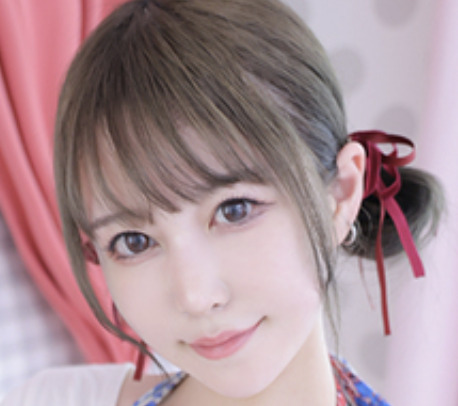
山口の絶対すぐ借りれる街金!
激甘審査でブラックでも借りれる街金、おすすめ17社。
お金が必要なのに、銀行や大手消費者金融の審査に通らない……
そんな悩みを抱えている方は多いのではないでしょうか。
特に、過去に金融事故がある方や、現在の収入が少ない方にとって、資金調達の選択肢は限られてしまいます。
しかし、山口には「激甘審査」の街金が存在し、一般的な金融機関よりも柔軟な審査基準で融資を受けられる可能性があります。
この記事では、山口の激甘審査の街金の特徴や、どのように利用すればスムーズに融資を受けられるのかを詳しく解説します。
また、総量規制や在籍確認の有無、即日融資の方法などについても触れ、利用者にとって有益な情報を提供します。
- 山口の激甘審査の街金!あなたを助ける公式サイトはこちら
- 1.クレジットカード現金化【プライムウォレット】選ばれ続ける超優良店
- 2.クレジットカード現金化【インパクト】最短5分で振込完了
- 3.クレジットカード現金化【あんしんクレジット】業界最高クラスの還元率
- 4.クレジットカード現金化【Quick現金サービス】とにかく早い!
- 5.クレジットカード現金化【どんなときもクレジット】初めての方、換金率3%UP
- 【QUICK39】お金を貸してくれるところをご紹介
- 1.【フクホー】ゆとりのローン!安心の金利7.30%〜
- 2.【スカイオフィスキャッシング】スピードと信頼の消費者金融
- 3.【キャレント】で借りんと!融資申込みキャンペーン中!
- 4.【アロー】最短即日融資もOK
- 5.【アルコシステム】信頼と実績のキャッシング
- 6.【プラン】全国どこからでも24時間簡単ご契約
- 7.【クレジットのニチデン】簡単・便利なネットキャッシング
- 8.【いつも】いつものキャッシング
- 9.借りやすくて返しやすい!キャッシング【フタバ】
- 10.来店不要・振込キャッシング【パーソナルクレジット セントラル】
- 11.安心できるパートナー【ハローハッピー】フリーローン
- 12.来店不要・振込キャッシング【女性専用 マイレディス】
- 女性専用!在宅で稼ぐ【FANZAノンアダルトチャットレディ募集】
- 山口の激甘審査の街金|サービス対象地域について
- 山口の激甘審査の街金を探すときの注意点
- 山口の激甘審査の街金は審査が甘い
- 山口の激甘審査の街金は在籍確認なしか
- 山口の激甘審査の街金は総量規制オーバーでも借りれるか?
- 山口の激甘審査の街金で即日融資を受けるには
- 山口の激甘審査の街金と、闇金との違い
- 山口の激甘審査の街金で審査落ち・・お金を借りれないときは
山口の激甘審査の街金!あなたを助ける公式サイトはこちら
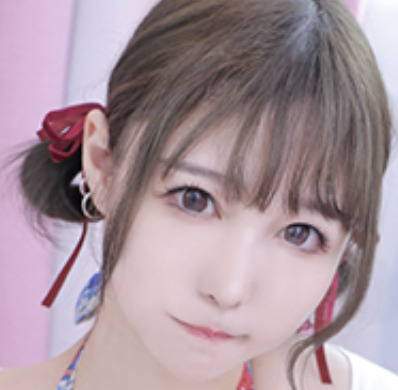
かんたんアンケートに答えるだけで、おこづかいをゲット
無料会員登録!アンケートに参加するだけで、お小遣い稼ぎ |
|||
サービス名 | サービス内容 | 報酬額 | 無料登録 |
【マクロミル】![]() |
スマホやPCで手軽にアンケートに答えて「現金」や「電子ギフト券」に交換可能なポイントがもらえます! | 新規登録で最大1000ポイント!(1,000円相当) | \会員120万人/![]() |
【オピニオンワールド】![]() |
かんたん登録1分、会員登録無料!ポイントを現金かギフトカードに交換 | アンケート1回につき、最高900円! | \900円ゲット/![]() |
【ボイスノート」】![]() |
かんたん登録1分、アンケート参加でポイントを現金かギフトカードに交換 | 会員登録だけで70ポイント獲得! | \今すぐ登録/![]() |
【モニタータウン![]() |
かんたん登録1分、設定5分、会員登録無料!現金かギフトカードに交換 | アプリインストールでポイント獲得(2,800円相当) | \iphone専用/![]() |
【モニタータウン![]() |
かんたん登録1分、設定5分、会員登録無料!現金かギフトカードに交換 | アプリインストールでポイント獲得(2,800円相当) | \Android専用/![]() |
【モニタータウン】![]() |
かんたん登録1分、設定5分、会員登録無料!現金かギフトカードに交換 | アプリインストールでポイント獲得(900円相当) | \PC専用/![]() |
【ハピタス】 ![]() |
お買い物やサービスの申し込みでポイントを貯めて、現金やギフト券に交換 | 会員登録時・500ptをプレゼント | \会員80万人/![]() |
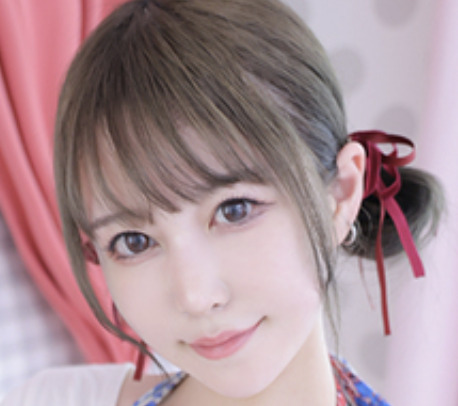
スマホと紹介コードが必須!
スマホで銀行口座を開設するだけでおこづかいゲット!
かんたん!【スマホ専用】無料の銀行口座開設でお小遣いをゲット | |||
銀行名 | 報酬額 | 紹介コード | 無料口座開設(スマホ必須) |
第一生命NEOBANK![]() |
¥1,500 |
oFFWZro |
第一生命NEOBANK![]() ![]() |
みんなの銀行![]() |
¥500 | hYBchqNL | みんなの銀行![]() ![]() |
UI銀行![]() |
¥500 | sh4402 | UI銀行![]() ![]() |
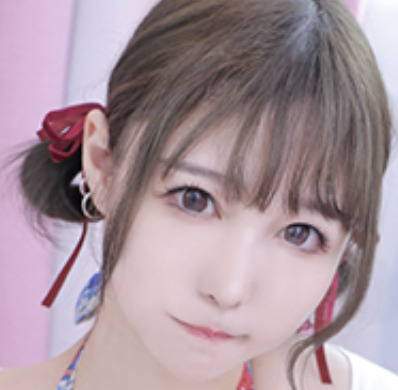
女性限定のお金稼ぎ!
在宅OKのかんたん美容モニタや、チャットレディ
【女性限定】かんたん!即金!お小遣い稼ぎができる在宅ワーク | |
【女性専用!美容モニター】![]() |
エステだけでなく、コスメや健康食品などを試せる在宅ワークのモニターも多数!「ファンモニ」(無料)会員登録はこちら 謝礼は1件1000円~5,000円ほど、複数組合わせれば月4万円以上も可能 |
【女性専用!在宅で稼ぐ】![]() |
自分の好きなタイミングで稼げるFANZAの在宅チャットレディ! ノンアダルトだから安心!スマホでかんたん在宅ワーク 時給7,500円以上可、安心の個人情報対策、最短翌日振込! |
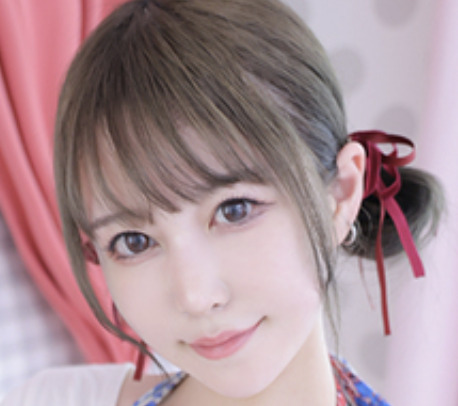
ファクタリングなら!
あなたの持っている債権を買取ります!
ファクタリング | 即日融資 | 手数料 | 申込み |
1.アクセルファクター![]() |
来店不要 最短2時間!即日融資 |
買取手数料0.5%~ 審査通過率 93.3%以上 |
\個人・法人/![]() |
2.資金調達プロ![]() |
かんたん10秒! 無料診断 最短即日融資 |
提携事業者数No1! 資金繰り改善率93%以上 |
\個人・法人/![]() |
3.株式会社No.1![]() |
最短30分で即日振込可能 | 買取手数料1%~(業界最安) 審査通過率 90%以上 |
\個人・法人/![]() |
4.エスコム![]() |
最短即日で審査完了。 即日に資金調達可能。 |
1.5%〜12% 資金化成功率97% |
\個人・法人/![]() |
5.Easy factor![]() |
最短10分でお見積り 最短60分で振込可能 |
2%〜8% 資金化成功率97% |
\個人・法人/![]() |
6.いーばんく![]() |
来店不要 最短即日入金可能 |
買取手数料4%~ 審査通過率 90%以上 |
\個人・法人/![]() |
7.ジャパンマネジメント![]() |
最短即日で審査完了。 翌日に資金調達可能。 |
三社間(3%~10%) 二社間(10%~20%) 資金化成功率93% |
\個人・法人/![]() |
8.PayToday![]() |
最短30分で審査完了。最短45分で振込可能。 | 1%〜9.5%(上限) | \個人・法人/![]() |
9.MSFJ![]() |
来店不要 最短1日で買取可能 |
1.8%〜9.8% 累積実績1万件以上 |
\個人・法人/![]() |
10.トラストゲートウェイ![]() |
来店不要 最短即日振込 |
1.5%〜9.5%(業界最安水準) 審査通過率95% |
\個人・法人/![]() |
11.トップ・マネジメント![]() |
来店不要 最短即日振込 |
3社間ファクタリング(買取対象債権の99.5%〜96.5%) 2社間ファクタリング(買取対象債権の96.5%〜87.5%) |
\個人・法人/![]() |
12.西日本ファクター![]() |
最短即日即買取り 診断無料 |
【九州、中国、四国エリアに限定】 手数料は2.8%〜 |
個人・法人(九州、中国、四国)![]() |
13.三共サービス![]() |
来店不要 資金化まで最短翌日 |
平均手数料8% 資金化成功率97% |
\法人のみ/![]() |
14.ネクストワン![]() |
来店不要 資金化まで最短即日 |
1.5%〜10%(業界最安水準) | \法人のみ/![]() |
15.ラボル![]() |
来店不要 最短30分入金 |
買取額の10% 振込手数料などの費用なし |
\個人事業主のみ/![]() |
16.請求書先払い![]() |
最短60分でお振込み 対面不要 |
手数料3%〜10% 最低買取金額10万円 |
\個人事業主のみ/![]() |
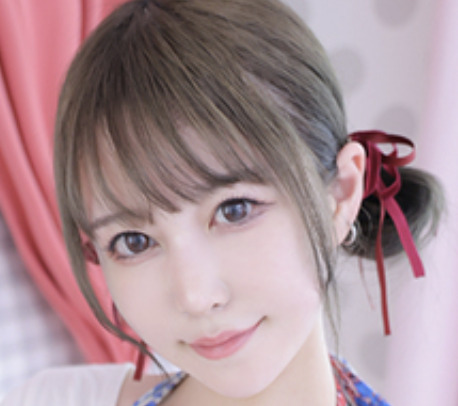
クレカの現金化サービスなら、即日にも対応してくれます!
社名 | 即日融資 | 実質年率 | 限度額 | 特徴 |
1.クレカ現金化「プライムウォレット」![]() |
最短10分 | 還元率最大98% | 設定なし | ・24時間受付 ・土日祝日も即日振込 ・カード事故過去0件 ![]() |
2.クレカ現金化「インパクト」![]() |
最短5分 | 還元率最大99.5% | 設定なし | ・審査不要 ・土日祝日も即日振込 ・来店不要 ![]() |
3.クレカ現金化「あんしんクレジット」![]() |
最短3分 | 還元率最大99.5% | 設定なし | ・審査不要 ・土日祝日も即日振込 ・来店不要 ![]() |
4.クレカ現金化「Quick現金サービス」![]() |
最短20分 | 還元率最大90%(手数料無料) | 設定なし | ・24時間365日受付 ・土日祝日も即日振込 ・審査不要 ![]() |
5.クレカ現金化「どんなときもクレジット」![]() |
最短5分 | 還元率最大98% | 設定なし | ・24時間受付 ・土日祝日も即日振込 ・スマホで申込完結 ![]() |
QUICK39のキャッシングローン紹介![]() |
お金を貸してくれるトコロを見つけます。 どこからも借りれない・・金利が高くもっと安いところへ借り換えしたい・・こんな悩みを解消します。 |
・完全無料 ・紹介料無料 ・手数料無料 ![]() |
||
1.フクホー![]() |
対応 | 7.3%〜18.0% | 1〜200万円 | ・24時間受付 ・来店不要 ・利息は利用日数分だけ ![]() |
2.スカイオフィスキャッシング![]() |
対応 | 15.0%〜20.0% | 1〜50万円 | ・24時間受付 ・来店不要 ・最短30分で審査完了 ![]() |
3.キャレント![]() |
対応 ※ | 7.8%〜18.0% | 1万円~500万円 | ・24時間受付 ・来店不要 ・申込まで3分で完了 ![]() |
4.アロー![]() |
対応 | 15.0%〜19.94% | 200万円まで | ・24時間受付 ・来店不要 ・かんたん3秒診断 ![]() |
5.アルコシステム![]() |
対応 | 15.0%〜20.0% | 1〜50万円 | ・24時間受付 ・来店不要 ・保証人・担保不要 ![]() |
6.プラン![]() |
対応 | 12.00%~18.00% | 1〜50万円 | ・24時間受付 ・来店不要 ・保証人・担保不要 ![]() |
7.クレジットのニチデン![]() |
対応 ※ | 7.3%〜17.52% | 1〜50万円 | ・24時間受付 ・来店不要 ・100日間利息なし(初回のみ) ![]() |
8.いつも![]() |
対応 | 4.8%~18.0% | 1万円~500万円 | ・24時間受付 ・来店不要 ・最大60日間利息なし(初回のみ) ![]() |
9.フタバ![]() |
対応 | 14.959%~19.945% | 1万円~50万円 | |
10.セントラル![]() |
対応 |
4.8%~18.0% |
1万円~300万円 | ・24時間受付 ・最大30日間利息なし(初回のみ) ・セブン銀行ATMでご利用ご返済が可能 ![]() |
11.ハローハッピー![]() |
対応 | 10.00%~18.00% | 100万円まで | ・24時間受付 ・来店不要 ・保証人・担保不要 ・パート・アルバイト・主婦の方も歓迎 ![]() |
12.マイレディス![]() |
対応 | 4.80%〜18.00% | 1万円〜100万円 | ・24時間受付 ・来店不要 ・保証人・担保不要 ・女性専用キャッシング ![]() |
【女性専用!在宅で稼ぐ】![]() |
自分の好きなタイミングで稼げるFANZAの在宅チャットレディ! ノンアダルトだから安心!スマホでかんたん在宅ワーク 時給7,500円以上可、安心の個人情報対策、最短翌日振込! |
|||
13.オージェイ![]() |
対応 | 9.5%〜18.0% | 10万円~5,000万円 | ・24時間受付 ・来店不要 ![]() |
14.アクト・ウィル![]() |
対応 | 7.5%〜15.0% | 300万円~1億万円 | ・24時間受付 ・来店不要 ・審査は最短60分 ![]() |
15.ファンドワン![]() |
対応 | 2.5%〜18.0% | 30万円~1億円 | ・24時間受付 ・来店不要 ・審査は最短40分 ![]() |
16.MRF![]() |
対応 | 4%〜15.0% | 30万円~3億円 | ・24時間受付 ・来店不要 ・24時間以内に仮審査 ![]() |
※ 平日14時までの手続完了が条件
激甘審査の絶対すぐ借りれる街金(消費者金融)をご紹介します。
どの消費者金融も独自審査を採用しており、柔軟な対応をしてくれるのでブラックでも借りれて即日融資OKの可能性が高いです。
ブラックだからと諦めずに真剣にお金が必要な理由を説明して少しでも融資をしてもらえる確率をあげましょう。
またクレジットカードを現金化してくれる優良サービス店も合わせてご紹介します。
1.クレジットカード現金化【プライムウォレット】選ばれ続ける超優良店
ショッピング枠現金化業界で超有名で誰もが知っているプライムウォレット!
現在日本でも、キャッシュレス化が進んでおりクレジットカードを保有する方は増えておりますね♪
15時以降でも即日振込み可能で、地方銀行もOKです。
2.クレジットカード現金化【インパクト】最短5分で振込完了
インパクトは、超高還元率(最高99.5%)でしられるクレジットカード現金化優良店です。
さらに新規申込で2%換金率UP!
審査不要、来店不要で最短5分でお振込み。
クレジットカードのショッピング枠があれば現金化してくれる安心の優良店です。
3.クレジットカード現金化【あんしんクレジット】業界最高クラスの還元率
あんしんクレジットは、超高還元率(最高98.8%)でしられるクレジットカード現金化優良店です。
審査不要、来店不要で最短5分でお振込み。
クレジットカードのショッピング枠があれば現金化してくれる安心の優良店です。
4.クレジットカード現金化【Quick現金サービス】とにかく早い!
Quick現金サービスは、振込金額を最初に提示する優良現金化サービスです。
24時間WEB対応やLINEチャットなど、お客様目線のサービスを提供し続けてリピーターは脅威の90%以上!
急な出費でお金が足りない!そんな方でも大丈夫!
5.クレジットカード現金化【どんなときもクレジット】初めての方、換金率3%UP
急な出費で困っていませんか?
どんなお悩みも、どんなときもクレジットにお任せください。
お申込みが初めての方なら換金率3%アップ!
審査不要、来店不要で今すぐ現金化してくれる安心サービスです。
【QUICK39】お金を貸してくれるところをご紹介
消費者金融からの借り入れが多数あってどこからも借りれないと思っている方や 金利が高くてもっと安いところへ借り換えしたい方・・・
このような悩みを抱えたお客様にたいして 安心して安全でより安く借り入れが出来るようにサポートさせていただいております。
完全無料ですのでお客様から紹介料・手数料等の料金は一切いただいておりません。
1.【フクホー】ゆとりのローン!安心の金利7.30%〜
★創業45年★
キャッシング・消費者金融のフクホー 安心の金利7.30%〜!
最高200万円までのゆとりのローン。
キャッシング・消費者金融・ローンなら創業45年のフクホー。
ご来店一切不要、振込み キャッシングの消費者金融です。
レディースキャッシングもご用意しているので、女性の方でも安心してご利用頂けます。
2.【スカイオフィスキャッシング】スピードと信頼の消費者金融
最短30分!来店不要 スピードキャッシング!!受付は24時間可能!
当日 9:00〜14:00 までにお申込み頂きますと、 即日のお振込みが可能です。
スカイオフィスニッセイキャッシングは他社で断られた方でも柔軟に対応している消費者金融です。
インターネットから申込みを行い、審査が完了すればその日に融資を受けられます。
来店不要のスピードキャッシングは急な出費でお困りの方の力強い味方です。
3.【キャレント】で借りんと!融資申込みキャンペーン中!
インターネット専用キャッシング♪完全非対面でネット完結。
カード発行不要!全国どこでもご指定の銀行口座にお振込み致します。
担保、保証人不要でキャッシングが可能です。
インターネット申込み、365日、24時間受付中!
申込フォームの入力内容が少なく、簡単にお手続きいただけます♪
ご利用限度額は【1万円〜500万円】まで。
大手消費者金融、カードローンの審査が通らなかった方でも柔軟な審査で対応致します。
4.【アロー】最短即日融資もOK
無担保かつスピーディーな使途自由ローンです。
・独自審査
・来店不要
・最短即日振込
・アプリならWEBで完結、郵送物は一切なし!
「郵送物が一切不要なアローWEB完結ローン」の取扱いを開始いたしました。
お申込み後、一次審査が通過した方は、スマホでアプリをダウウンロードして頂くことで、 サービスをご利用いただくことができます。
5.【アルコシステム】信頼と実績のキャッシング
創業1983年、信頼と実積、振込キャッシングの老舗です。
お申し込み後、最短でその日のうちにご指定口座に送金いたします。
貸付利率(実質年率) 年3.00%~20.00%
保証人・担保不要。
ご融資範囲 50万円まで(要審査)
お申し込みはWEBで、24時間受付中。
6.【プラン】全国どこからでも24時間簡単ご契約
全国どこからでも24時間ネットでお申し込みからご契約まで可能です。
又、キャッシュサービス『プラン』では1万円から最高50万円までご融資可能です。
・ご来店不要
・最短即日ご融資可能
・担保・保証人不要
・月々2,000円からのご返済も可能
7.【クレジットのニチデン】簡単・便利なネットキャッシング
簡単・便利・ネットキャッシング ニチデンのネットキャッシングへようこそ。
振込ローン・不動産担保ローン・事業者ローンをご用意しています。
学生ローンにも対応、20歳以上で定期的に収入のある方を対象としております(パート・アルバイト・主婦の方も歓迎します)
大学生〜幅広い層に対応しており、福山、福山と3店舗ありますので手厚いサービスをモットーとして運営しています。
8.【いつも】いつものキャッシング
『早い』 来店不要!24時間申込受付、最短45分でお振込完了。
『頼れる』ご利用は1万円〜最大500万円まで。
『便利』 カード発行不要!郵送物一切なし!お客さまに合わせた柔軟な在籍確認。
『安心』 LINEやメール・電話でご対応、ご融資まで完全サポート。
『納得』 電話応対コンクールにて数度入賞した丁寧なご対応。
9.借りやすくて返しやすい!キャッシング【フタバ】
創業50年以上の実績と信頼のキャッシングのフタバです。
・来店不要・即日振込みキャッシングです。
・インターネット申込みで24時間全国受付
・お申込みはとっても簡単、お申込みフォームに入力するだけ。
・過去に自己破産・債務整理等された方もまずはお申込みください。
・大手消費者金融やカードローンの審査が通らなかった方もお申込みください。
・女性スタッフが対応致します。
10.来店不要・振込キャッシング【パーソナルクレジット セントラル】
<<創業40年を超える安心と実績の「セントラル」>>
WEB申込で当日最短融資可能です!
全国の皆さまからのお申込みに対応いたします。
セントラルは昭和48年創業の老舗の貸金業です。
全国に17店舗展開しております。 東京都内4店舗(浅草、新橋、新宿、池袋)、埼玉と神奈川に各1店舗 愛媛6店舗、香川2店舗、高知1店舗、岡山2店舗 計17店舗展開。
全国のセブン銀行ATM提携を開始し、 全国の方々にもますますご利用していただきやすくなりました。
さらに、ご利用が初めての方は最大30日間金利0円!
11.安心できるパートナー【ハローハッピー】フリーローン
ハローハッピーは身近なキャッシング!
20歳以上で定期的に収入のある方を対象としております(パート・アルバイト・主婦の方も歓迎します)
家電ローン:家電製品のご購入
教育ローン:保育園〜大学の学費
リフォームローン:ご自宅の増改築・修理
医療ローン:入院、通院費 事業資金ローン
事業資金ローン:自営業、学資資金
ハローハッピーは各都道府県の方々へのサービスが手厚いお店です。
12.来店不要・振込キャッシング【女性専用 マイレディス】
<<創業40年を超える安心と実績の「セントラル」>>
そんなセントラルから生まれた女性専用のキャッシング【マイレディス】
WEB申込で当日最短融資可能です!
全国の皆さまからのお申込みに対応いたします。
中四国中心に店舗展開の老舗セントラルは、首都圏店舗も拡充中!
全国のセブン銀行ATM提携を開始し、 全国の方々にもますますご利用していただきやすくなりました。
さらに、ご利用が初めての方は最大30日間金利0円!
女性専用!在宅で稼ぐ【FANZAノンアダルトチャットレディ募集】
自分の好きなタイミングで稼げる在宅チャットレディ。
ノンアダルトだから安心、スマホで出来るかんたん在宅ワークです。
初めての方でも「業界最大手」「FANZAだから安心」してお仕事できます。
高収入在宅アルバイトならFANZAチャットガール!
隙間のお時間でお仕事可能、副業としても大歓迎です。
万全の個人情報管理、時給7,500円以上可、最短翌日振込!
山口の激甘審査の街金|サービス対象地域について
山口県でお金に困ったとき、銀行や大手の金融機関では審査が通らなかった…という経験を持つ方も少なくありません。そんなときに頼りになるのが、柔軟な対応で知られる街金(消費者金融)です。特に「激甘審査」と呼ばれる街金(消費者金融)は、他で断られた方でも利用できるチャンスが高く、地元住民を中心に需要が広がっています。
ここでは、山口県内で激甘審査に対応する街金(消費者金融)の特徴や、どの地域でサービスが受けられるのかを、わかりやすく解説していきます。
激甘審査とは?やさしく説明します
まず、「激甘審査」という言葉が気になる方も多いでしょう。
これは、他の金融機関と比べて審査がゆるやかで、過去に金融事故歴(延滞、債務整理、自己破産など)があっても、一定の条件を満たしていれば融資を受けられる可能性が高いことを指します。
もちろん誰でも無条件で借りられるというわけではありませんが、以下のような方には特に利用しやすい傾向があります。
-
他社で断られた経験がある
-
パートやアルバイトで収入が安定していない
-
クレジットカードやローンの延滞履歴がある
-
一度債務整理をしたことがある
街金(消費者金融)はこういった事情をふまえて、申込者の現状や生活状況を柔軟に判断してくれるため、銀行よりも「人間らしい」審査とも言われています。
山口県で利用できる街金(消費者金融)の特徴
山口県には都市部と地方部がバランスよく存在しており、それぞれの地域で特色のある街金(消費者金融)が営業しています。特に、地元に根ざした中小規模の街金(消費者金融)では、地域事情に詳しく、相談しやすいというメリットがあります。
また、最近ではインターネットからの申込みや電話対応も積極的に行われており、離島部や山間部に住んでいる方でも不便なく利用できます。
地域別|山口県内のサービス対象エリア
下関市エリア
山口県の西の玄関口である下関市には、古くからの金融業者が多く存在しています。
特に、地元密着型の街金(消費者金融)は、「人柄重視」の審査スタイルをとることがあり、収入証明書が簡易でも相談に応じてくれるケースもあります。
下関駅周辺には来店型の事業所もあり、顔を合わせて相談できる安心感があります。
宇部市・山陽小野田市エリア
工業都市として知られる宇部市と山陽小野田市では、製造業に従事する方や日雇い・派遣労働者の方に対応した街金(消費者金融)の需要があります。
この地域では、収入の変動がある方でも、過去の勤務年数や生活スタイルから融資の可否を判断してくれる業者が多く、激甘審査の傾向が強いとされています。
山口市・防府市エリア
山口市は県庁所在地として行政機能が集中しており、街金(消費者金融)も多様な業種の方を対象にしています。
特に公務員や契約社員、年金受給者などに対しても融資相談がしやすい環境があります。
また、防府市では、小規模な事業を営む個人商店主の方などが利用しやすい街金(消費者金融)が点在しており、事業資金の少額融資にも柔軟に対応してくれることがあります。
岩国市・柳井市・周南市エリア
山口県東部に位置するこれらの都市では、地元の交通事情や勤務状況を把握した街金(消費者金融)が多く、車通勤の人や単身赴任者にも配慮した対応をしています。
たとえば、来店が難しい方向けに郵送契約やWeb契約に対応している業者もあり、地方ならではの距離的な不利をカバーしてくれる配慮がされています。
萩市・長門市などの山間部
山口県の北部にあるこれらの地域では、街金(消費者金融)自体の数は少ないものの、オンライン申込や電話相談による融資対応を行っている業者がサポートしてくれます。
顔が見えにくいからこそ、審査内容に不安を感じる方もいますが、事前にきちんと相談することで、誠実な対応をしてくれる業者を見つけることができます。
山口県内の街金(消費者金融)を使うときの注意点
激甘審査とはいえ、安心して利用するためにはいくつかのポイントを押さえておくことが大切です。
1. 正確な情報で申し込む
虚偽の申告は、どんなに審査がやさしい業者でも契約が成立しません。住所や職業、収入などは正確に伝えましょう。
2. 無理な借入はしない
借りられるからといって、返済できない額を借りるのはリスクがあります。街金(消費者金融)は比較的少額からでも借りられるので、必要な分だけ借りるようにしましょう。
3. 契約内容を必ず確認
契約書の内容や金利、返済スケジュールなどは、しっかり読んでからサインするようにしましょう。不明な点があれば遠慮せずに質問しましょう。
山口県での街金(消費者金融)利用が選ばれる理由
山口県の街金(消費者金融)は、大手に比べて「顔が見える対応」「柔軟な審査」「地元に寄り添ったサービス」が魅力です。
急な入院、車の修理費、冠婚葬祭など、生活の中で突然発生する出費に対して、頼れる存在として活用されています。
また、審査に通る可能性が高く、スピードも早いため、銀行ローンを断られた経験がある方や信用情報に不安がある方にも心強い選択肢となります。
山口の激甘審査の街金を探すときの注意点
急な出費や予期しない状況でお金が必要になることはよくあります。
そんなときに頼りになるのが「街金」、つまり消費者金融です。特に山口では、激甘審査を掲げる街金も多く、誰でも利用しやすい環境が整っています。しかし、どんなに審査が甘いといっても、利用する際にはいくつかの注意点があります。今回は、山口の激甘審査の街金を探すときに気をつけるべきポイントについて詳しく説明します。
1. 審査が甘い理由を理解する
街金の多くは、審査が緩やかなことで知られています。これは、借り手の信用情報に厳密に依存しないケースが多いためです。
しかし、このような甘い審査の裏には、いくつかのリスクが隠れていることもあります。
高金利に注意
審査が甘い分、金利が高めに設定されていることが多いです。
これにより、借りたお金を返済する際に想像以上の負担がかかる可能性があります。金利が高いと、返済総額が大きく膨らむため、借り入れ前にしっかりと金利を確認することが重要です。
過剰融資に注意
激甘審査を掲げる街金では、簡単に融資を受けられる一方で、過剰融資をしてしまうリスクもあります。
借りる金額を自分でしっかり管理し、返済能力を超えない範囲で利用することが求められます。借りすぎは後々の負担となり、返済が困難になる可能性があるので、慎重に計画を立てる必要があります。
2. 事前に利息・手数料をしっかり確認する
街金の利用者がしばしば見落としがちなのが、利息や手数料に関する詳細な確認です。
金利だけでなく、事務手数料やATM手数料など、他の費用がかかる場合があります。
金利の計算方法を理解する
金利の計算方法には、「日割り金利」や「年率」などの種類があります。
日割り金利では、借りた日数に応じて利息が計算されるため、借りる期間が長ければ長いほど金利負担が増えることになります。金利の計算方法を理解し、できるだけ短期間で返済できるように計画しましょう。
その他の手数料
一部の街金では、契約時に事務手数料を請求することがあります。
また、ATMで現金を引き出す際に、提携ATM以外を使用すると手数料が発生する場合があります。これらの手数料を事前に把握し、余計な費用を避けるように心がけましょう。
3. 返済能力をしっかり確認する
街金を利用する際に最も重要なのが、返済能力の確認です。
利用額を決定する前に、自分の収入や支出をしっかりと見直し、月々の返済が可能かどうかを検討する必要があります。
返済計画を立てる
お金を借りる前に、返済計画を立てることは非常に大切です。
月々の返済額や返済期間をしっかりと計算し、無理なく返済できる額を借りるようにしましょう。急な出費があっても、余裕を持って返済を続けられるようにするため、計画的に借り入れを行いましょう。
収入の安定性を考慮する
収入の安定性も返済能力に大きく関わります。
もし収入が不安定であれば、返済が困難になる可能性が高いため、安定した収入が見込める仕事をしているかどうかを再確認しましょう。また、将来的な支出の増加が見込まれる場合には、借入額を抑えることをおすすめします。
4. オンライン融資の利用を検討する
最近では、多くの街金がオンライン融資を提供しています。
オンラインでの融資申し込みは、時間がない人や店舗に足を運びたくない人にとって非常に便利です。ただし、オンライン融資を利用する際にもいくつかの注意点があります。
安全性を確認する
オンラインでお金を借りる際は、特に安全性に注意が必要です。
信頼性のある金融機関かどうかを見極めるために、公式サイトの情報や口コミを確認しましょう。また、個人情報の取り扱いについても注意を払い、安全なサイトで申し込むようにしましょう。
即日融資の可能性
オンライン融資は、多くの場合、即日融資が可能です。
しかし、審査のスピードや融資額によっては、即日融資を受けることができない場合もあります。急いでいる場合は、即日融資が可能かどうかを事前に確認しておくと良いでしょう。
5. 無理な借り入れを避ける
「街金を使えば簡単にお金が借りられる」という考えが浮かぶこともあるかもしれませんが、無理に借り入れを行うことは後々大きな負担になります。
借り入れは、あくまで必要な時に、必要な金額だけにしましょう。
借りる前に再確認
「本当にお金が必要なのか」を再確認し、他にお金を調達する方法がないかを検討してください。
家族や友人からの支援や、貯金を取り崩すなど、他の方法で対応できる場合もあります。無理に街金を利用する前に、他の選択肢を考えることも重要です。
山口には、審査が甘く、スピーディーに融資が受けられる街金が多くありますが、利用に際しては慎重に選び、条件をしっかりと確認することが重要です。
金利や手数料、返済計画を事前に確認し、無理のない借り入れを心がけましょう。急な資金調達が必要な場合でも、冷静に判断し、自分の生活に支障をきたさないように利用することが大切です。
山口の激甘審査の街金は審査が甘い
山口には、急な資金需要に対応するために、非常に甘い審査基準を設けている街金(消費者金融)が多数存在しています。
激甘審査の街金は、通常の金融機関よりも柔軟な審査を行っているため、借りやすいという特徴があります。しかし、この甘い審査基準には注意すべき点も多いため、しっかり理解して利用することが重要です。
今回は、山口の激甘審査の街金の特徴、審査基準、メリットとデメリット、そして利用する際の注意点について詳しく解説します。
1. 激甘審査の街金の特徴
激甘審査の街金は、銀行や大手消費者金融と比べて、融資基準が緩いため、多くの人が利用しやすいという特徴があります。
審査基準が甘いとはいえ、無条件で融資を受けられるわけではありませんが、一般的には以下の点が特徴として挙げられます。
・低い信用スコアでも審査が通る
大手の消費者金融では、一定の信用スコアや安定した収入を求められますが、激甘審査の街金は、これらの基準をかなり緩く設定しています。
過去に金融事故を起こした人や、信用情報に問題がある人でも審査に通ることがあります。
・即日融資が可能
激甘審査を行っている街金の中には、申し込みから審査、融資までを迅速に行っているところも多くあります。
急いでお金を借りたいという場合でも、即日融資が可能なことがあるため、非常に便利です。
・柔軟な審査基準
山口の激甘審査の街金では、年齢や職業などに関しても比較的寛容な審査を行っています。
例えば、アルバイトやフリーランスなど、安定した収入がなくても借りられる場合があります。
2. 審査が甘い理由とは?
なぜ山口の街金は他の消費者金融と比べて審査が甘いのでしょうか?
その理由をいくつか挙げてみます。
・小規模な運営でリスクを取る
激甘審査を行っている街金は、大手消費者金融とは異なり、規模が小さく、独自の融資基準を設けていることが多いです。
規模が小さいため、リスクを取って融資を行うことができ、審査基準を緩くして多くの借り手に融資を提供しています。
・金利でリスクをカバー
甘い審査を行うことで、借り手が多くなり、貸し倒れのリスクも高くなります。
しかし、激甘審査を行っている街金は、金利を高めに設定していることが多いです。この高金利によって、貸し倒れのリスクをカバーし、安定した利益を得ています。
・迅速な審査と融資で集客
街金の多くは、迅速に融資を行うことを売りにしており、そのため審査もスピーディーです。
審査が早ければ、利用者の利便性が向上し、集客につながるため、多少甘い審査基準を設けることができます。
3. 激甘審査の街金を利用するメリット
激甘審査の街金を利用することで、どのようなメリットがあるのでしょうか。
代表的なメリットをいくつか紹介します。
・スピーディーな対応
急にお金が必要になった場合、激甘審査の街金は即日融資を行っていることが多いため、スピーディーにお金を手に入れることができます。
特に、銀行などの審査に時間がかかる場合に比べて、圧倒的に早く融資が受けられる点は大きな魅力です。
・低い審査基準
大手の消費者金融や銀行では、一定の信用スコアや収入の安定性が求められますが、激甘審査の街金は比較的低い基準で融資を行っています。
そのため、他の金融機関で審査に通らなかった人でも、融資を受けやすいという利点があります。
・審査が柔軟
収入の安定性や職業に関しても柔軟な対応をしているため、アルバイトやフリーランスなど、安定収入がない場合でも融資が受けられることがあります。
4. 激甘審査の街金を利用するデメリット
一方で、激甘審査を利用することにはデメリットもあります。
甘い審査基準が裏目に出ることもあるため、注意が必要です。
・高金利
激甘審査の街金は、金利が高めに設定されていることが多いです。
金利が高いため、借りた金額を返済する際には、利息が膨らんでしまうことがあります。長期間にわたって返済を続ける場合、利息だけでかなりの額を支払うことになる可能性があります。
・過剰借り入れのリスク
審査が甘いため、つい必要以上にお金を借りてしまうことがあります。
その結果、返済が困難になり、借金の連鎖に陥るリスクがあります。特に、金利が高い場合は、返済額が膨らんでしまい、生活が困窮する恐れがあります。
・貸し倒れリスク
激甘審査を行っている街金は、信用情報に問題がある人にも融資を行っているため、貸し倒れリスクが高くなります。
もし返済ができなかった場合、高い金利や追加費用がかかり、借金が膨らんでしまうことがあります。
5. 注意すべきポイント
激甘審査の街金を利用する際には、以下の点に注意することが重要です。
- 借入額を必要最小限に抑える: 必要以上にお金を借りないようにしましょう。無理に借りすぎると、後で返済が困難になります。
- 金利の確認: 金利が高いことが多いので、事前に金利をしっかりと確認し、返済計画を立ててから借り入れることが重要です。
- 返済計画を立てる: 返済能力に応じた計画を立て、無理のない返済を心がけましょう。
激甘審査の街金を賢く利用するためには、これらのポイントを意識して行動することが必要です。
山口の激甘審査の街金は、審査が甘いため、借りやすいという魅力がありますが、同時に高金利や過剰借り入れのリスクも伴います。
しっかりと返済計画を立てて、借りすぎないように注意し、利息や契約内容をしっかり理解してから利用することが大切です。
山口の激甘審査の街金は在籍確認なしか
山口で街金(消費者金融)を利用する際、よく気になるのが「在籍確認」の有無です。
在籍確認は、申込み時に提出した勤務先の情報が正しいかどうかを確認するためのプロセスで、融資を受けるためには避けて通れない重要なステップの一つです。しかし、なかには在籍確認を行わない街金もあります。この記事では、山口の激甘審査の街金が在籍確認を行うのか、またそれがどういった意味を持つのかについて詳しく解説します。
在籍確認とは?
在籍確認とは、借入の申込みを行う際に、申込者が記載した勤務先に対して、実際にその場所で働いているかどうかを確認する作業です。
この確認が行われる理由は、貸し手が借り手の返済能力を確かめるためです。申込者が申告した勤務先で実際に働いていない場合、返済能力に疑問を持たれることになります。
在籍確認は基本的に電話で行われることが多いですが、最近では、勤務先に電話をかけずに、給与明細書などの書類を提出することで在籍確認を代替する場合もあります。
山口の街金では在籍確認をしないことがある
山口の街金(消費者金融)の中には、在籍確認を行わないところもあります。
特に激甘審査を売りにしている街金では、審査を通過させるために、利用者の負担を軽減するために在籍確認を省略することがあるのです。
このような街金が在籍確認を行わない理由として、以下の点が挙げられます。
1. 少額融資のため
街金は、一般的に少額の融資を提供しています。少額の融資であれば、在籍確認を省略してもリスクが低いと見なされることが多いです。
例えば、数万円程度の融資であれば、借り手の返済能力があるかどうかを見極めるために在籍確認を行わなくても十分だと考えるのです。
2. スピーディーな審査を重視
激甘審査の街金は、迅速に融資を行うことを優先しています。
そのため、審査にかかる時間を短縮するために、在籍確認を省略する場合があります。利用者にとっても、即日融資が可能になるため、非常に便利なサービスです。
3. 書類確認で代替できる場合がある
街金によっては、在籍確認を電話ではなく、給与明細書や源泉徴収票などの書類で代替することができます。
これにより、利用者が職場に電話される心配を減らすことができ、在籍確認を避けることができるのです。
在籍確認が行われる場合の対応方法
しかし、全ての街金が在籍確認を行わないわけではありません。
中には、在籍確認を必ず行う街金も存在します。もし在籍確認が必要な場合でも、以下の方法で対応することができます。
1. 自分で担当者に相談する
在籍確認を避けたい場合、申し込む前に街金に確認しておくことが重要です。
多くの街金は、申込者の事情を考慮し、柔軟に対応してくれることがあります。例えば、職場に電話されることを避けたい場合、事前に「勤務先に連絡しないでほしい」とお願いすることができる場合があります。
2. 代替書類を提出する
在籍確認を避けたい場合、給与明細書や源泉徴収票などの書類を提出することで、電話での確認を回避できることがあります。
これにより、職場に直接電話される心配がなく、プライバシーが守られます。提出する書類については、事前に街金に確認をしておくと良いでしょう。
3. 家族や友人に協力してもらう
在籍確認をどうしても避けなければならない場合、家族や友人に協力してもらう方法もあります。
例えば、家族や友人に勤務先の情報を伝え、在籍確認を依頼することが可能です。ただし、これが必ず許可されるわけではなく、街金の方針により異なるため、事前に確認が必要です。
在籍確認がない場合のリスクと注意点
街金で在籍確認が行われない場合、利用者にとっては便利である一方で、いくつかのリスクもあります。
1. 高い金利
在籍確認がない場合、金利が高く設定されていることが多いです。
これは、貸し手側がリスクを軽減するために、金利でそのリスクをカバーしようとしているためです。急な資金需要に応えるために利用する場合、金利が高くても許容できる場合が多いですが、長期的に借りる場合には注意が必要です。
2. 利用額の制限
在籍確認が行われないことにより、融資額が制限される場合があります。
少額融資が主流となり、返済能力を見極めるための詳細な審査が行われないため、貸し出せる金額に限度があるのです。大きな金額を借りたい場合には、他の金融機関に頼る方が良いこともあります。
3. 返済能力が疑われる場合がある
在籍確認を行わない場合、申込者の返済能力が不明瞭になることがあります。
そのため、返済に関する厳しい審査が行われる可能性があります。街金側が審査を軽視することなく、貸し付ける金額を慎重に決めることが重要です。
山口の激甘審査の街金は総量規制オーバーでも借りれるか?
山口の街金(消費者金融)を利用したいと考えるとき、よく耳にするのが「総量規制」という言葉です。
総量規制とは、借り入れできる金額が年収の3分の1までに制限されるというルールですが、この制限を超えて借りたい場合、どうすればよいのでしょうか?
今回は、総量規制オーバーでも山口の街金で融資を受けられるのかについて、わかりやすく説明します。
総量規制とは?
総量規制とは、貸金業法によって定められた規制で、消費者金融を利用して借りられる金額が、借り手の年収の3分の1までに制限されるというルールです。
この規制の目的は、過剰な借入れを防ぎ、借り手が返済困難な状況に陥るのを避けることです。
例えば、年収が300万円の人であれば、最大で100万円までの借入れしかできません。これを超えて借り入れを行うことは、法律上禁止されています。
山口の街金は総量規制を守っている?
山口の街金(消費者金融)は、原則として総量規制に従って融資を行っています。
つまり、年収の3分の1以上の借り入れを希望しても、その金額を超えて貸し付けることはできません。
しかし、街金によっては、少しでも柔軟に対応してくれるところもあります。例えば、少額の借り入れや一時的な借り入れに関して、条件を緩和することがあるかもしれませんが、総量規制を完全に無視することはありません。
総量規制オーバーでも借りる方法
では、総量規制を超えた借入れをしたい場合、どうすればよいのでしょうか?
ここでは、総量規制オーバーでも借りるための方法について、いくつかのポイントを紹介します。
1. 返済中の借り入れを減らす
総量規制オーバーの原因のひとつに、すでに他の消費者金融から借り入れをしていることが挙げられます。
すでに他社で借り入れがある場合、その借入金額が年収の3分の1を超えてしまっていることが多いです。この場合、他の借入れを完済するか、返済額を減らすことで、新たに借り入れができるようになります。
2. 他の貸金業者を利用する
街金(消費者金融)の中には、総量規制を遵守しつつも、他の貸金業者とは異なる基準で審査を行っているところもあります。
例えば、特定の業者は、既存の借り入れが他社でのものであれば、審査が厳しくなることが少ない場合もあります。また、一定の信用を築くことができれば、借り入れ額が増えることもあります。
3. 配偶者の収入を合算する
総量規制では、あくまで借り手本人の年収が基準となりますが、配偶者の収入を合算して審査を行ってくれる場合もあります。
これを「配偶者貸付け」と呼びます。もし配偶者が働いている場合、その収入を加算して借り入れの上限額を増やすことができることがあります。
4. 借り換えを利用する
総量規制オーバーの状態であっても、借り換えを利用することで、新たに融資を受けられる場合があります。
借り換えとは、現在の借り入れを他の消費者金融でまとめることで、1本化し、金利の低減や返済額の軽減を図るものです。この際に、他の借り入れが減ることで、総量規制をクリアできる可能性もあります。
山口の街金で借りる際の注意点
総量規制オーバーでも借り入れができる場合がありますが、注意すべき点もいくつかあります。
1. 高金利の可能性
総量規制オーバーで借り入れをする場合、貸金業者は融資リスクが増すため、高い金利が設定される可能性があります。
高金利での借り入れは、返済額が増え、最終的に支払いが難しくなるリスクが高くなります。そのため、必要な金額だけを借りるようにし、返済計画をしっかり立てることが重要です。
2. 審査が厳しくなる
総量規制オーバーの状態で借りるためには、審査が厳しくなることが一般的です。
多くの消費者金融では、すでに借り入れがある人に対して、慎重な審査を行います。そのため、過去の返済履歴や信用情報に問題がないことが求められます。
3. 返済計画の重要性
総量規制オーバーで借り入れを行う場合、返済計画をしっかりと立てることが必須です。
特に高金利で借りる場合、返済が困難にならないようにするために、無理のない範囲で借り入れを行うようにしましょう。
山口の激甘審査の街金で即日融資を受けるには
山口で急にお金が必要になったとき、街金(消費者金融)の即日融資が頼りになることがあります。
特に「激甘審査」で知られる山口の街金では、審査がスムーズに進むことが多く、迅速に融資を受けられる可能性が高いです。
しかし、即日融資を受けるためには、いくつかの注意点や条件を理解しておくことが重要です。
この記事では、山口の街金で即日融資を受けるための方法を、やさしく、詳しく、わかりやすく説明します。
1. 即日融資の基本条件
即日融資を受けるためには、いくつかの基本的な条件をクリアする必要があります。
これらの条件は、審査のスピードを左右する重要なポイントとなるので、しっかり理解しておきましょう。
1.1 審査が迅速であること
街金(消費者金融)では、通常、即日融資を行っているところが多いですが、そのためには審査が迅速に行われる必要があります。
即日融資を希望する場合、審査結果がすぐに出るように、以下の条件を整えておくことが大切です。
1.2 必要書類を事前に準備する
審査のスピードを上げるためには、事前に必要な書類を準備しておくことが不可欠です。
以下の書類は、ほとんどの街金で必要とされます。
- 本人確認書類(運転免許証、パスポート、健康保険証など)
- 収入証明書(給与明細、税務署からの納税証明書、確定申告書など)
- 住所確認書類(公共料金の領収書など)
これらの書類を事前に用意し、オンライン申し込み時にアップロードできるようにしておくと、審査がスムーズに進みます。
2. 即日融資を受けるための申し込みの流れ
街金で即日融資を受けるためには、申し込みから融資実行までの流れを把握しておくことが重要です。
以下は、一般的な申し込みの流れです。
2.1 オンラインで申し込む
ほとんどの街金では、インターネットから申し込みができるオンライン申し込みシステムを提供しています。
オンラインで申し込むことで、店頭に出向く手間を省けるうえ、審査結果も早く届きます。申し込み時には、上記の必要書類をデジタルで提出することが求められるので、事前に準備しておきましょう。
2.2 審査を受ける
申し込みが完了すると、街金の担当者が審査を開始します。
審査は、信用情報、収入、借入履歴などをもとに行われます。山口の激甘審査の街金では、比較的柔軟な対応をしているところが多く、過去に問題があった人でも審査に通る場合があります。
審査結果は通常、数分から30分程度で届きます。もし審査に通った場合は、融資金額や金利、返済方法などの条件が提示されます。
2.3 融資実行
審査に通った後、融資実行が行われます。
即日融資を受けるためには、営業終了前に融資が実行される必要があります。街金によっては、営業時間が長く、夜遅くまで融資を行っているところもあります。
一般的には、午後3時から4時までに申し込むと、即日融資が受けやすいです。
融資方法には、銀行口座への振り込み、ATMでの受け取り、または店舗での現金受け取りなどがあります。振り込みを選ぶ場合は、振り込み時間に注意する必要があります。特に、金融機関の営業時間内に振り込まれなければ、翌営業日に繰り越されてしまうこともあります。
3. 即日融資を受けるためのポイント
即日融資をスムーズに受けるためには、いくつかのポイントを押さえておくことが重要です。
以下の点に注意することで、融資のスピードが早まる可能性があります。
3.1 申し込み時間を早めに設定する
即日融資を希望する場合、申し込みを早めに行うことが大切です。
街金によっては、午後3時以降の申し込みでは、即日融資が受けられない場合もあります。できるだけ早い時間に申し込みを済ませ、余裕を持った対応をしましょう。
3.2 審査を早く通過するために信用情報を整える
街金の審査は信用情報を重視しています。
そのため、信用情報が悪いと審査に通らない可能性があります。もし過去に延滞や債務整理を行ったことがある場合、その情報が残っているかもしれません。審査を通過するためには、できるだけ早く信用情報を整理しておくことが大切です。
3.3 必要書類は迅速に提出する
オンライン申し込みでは、必要書類の提出が求められます。
書類の提出を遅延させると、審査が遅れる原因になります。提出を迅速に行うことで、融資の審査が早く終わり、即日融資を受けることが可能となります。
4. 山口の街金の選び方
山口には多くの街金(消費者金融)があり、それぞれが異なるサービスを提供しています。
即日融資を受けるためには、融資スピードや審査の柔軟さ、金利などを比較して、自分に合った街金を選ぶことが重要です。
- 審査スピードの速さ: 即日融資を希望する場合、審査スピードの速さを確認しておきましょう。
- 金利の確認: 融資を受ける際には金利が重要なポイントです。即日融資を希望する場合でも、金利が高すぎないかを確認することが大切です。
- 融資限度額や返済方法: 自分が必要とする融資額に合った街金を選び、返済方法が自分の生活スタイルに合ったものを選びましょう。
5. 注意点
即日融資を受ける場合、返済計画がしっかりしていないと後々大変なことになりかねません。
返済を遅れた場合、追加で利息が発生したり、信用情報に傷がついたりすることがあります。借り入れは慎重に行い、返済計画をしっかりと立ててから融資を受けるようにしましょう。
山口の激甘審査の街金と、闇金との違い
お金を借りるとき、選択肢として「街金(消費者金融)」と「闇金」という言葉がよく登場します。
しかし、これらは一見似ているようで、実際は大きな違いがあります。
特に山口には、激甘審査を提供する街金が存在し、急な融資を希望する人々にとって非常に便利なサービスを提供していますが、闇金とは異なる点が多いです。
1. 街金(消費者金融)とは?
街金とは、消費者金融業者が提供するサービスで、一般的に個人向けの融資を行っています。
これらの業者は、法律に基づいて運営されており、消費者保護を考慮したサービスを提供しています。
1.1 正規の金融業者
街金は、金融庁に登録された正規の金融業者です。
したがって、法的な規制の下で運営され、金利や融資条件についても法律に則って設定されています。融資金額や返済期間などが事前に決まっており、利用者は契約内容をしっかりと確認することが求められます。
1.2 激甘審査
山口の激甘審査を特徴とする街金は、他の金融機関よりも柔軟に審査を行います。
これにより、収入が不安定な人や信用情報に問題がある人でも融資を受けられることがあります。審査基準が甘いため、融資を希望する人々にとっては非常に便利なサービスですが、同時に注意が必要です。
1.3 金利や返済計画の透明性
街金では、金利や返済方法について透明性が保たれています。
金利は法律で上限が決まっており、過剰な金利を請求されることはありません。また、返済期日や方法についても契約時に詳細な説明があり、利用者は不安なく利用することができます。
2. 闇金とは?
闇金とは、無登録で不正に営業している金融業者のことを指します。
これらの業者は、法律に基づかず、違法な方法でお金を貸し付けているため、大きなリスクが伴います。
2.1 無登録・違法な業者
闇金業者は、金融庁や警察に登録されていない業者であり、違法に営業を行っています。
彼らは通常、無許可で融資を行い、利息の上限を超える高金利を設定することが多いため、借り手にとって非常に危険です。
2.2 過剰な金利と罠
闇金業者は、金利を法定金利の何倍にも設定し、借り手に過剰な返済を強いることが一般的です。
場合によっては、借金が雪だるま式に膨らむこともあります。金利が異常に高いため、返済が追いつかず、借り手がさらなる借り入れをするよう仕向けられることもあります。
2.3 不透明な契約内容
闇金との契約には、詳細な説明がないことが多く、借り手が知らない間に契約内容が変更されたり、返済条件が厳しくなることがあります。
最初は簡単にお金を借りられるように見えますが、後々大きな問題になることがよくあります。
3. 街金と闇金の違い
街金と闇金の大きな違いは、法的な正当性と貸し付け条件にあります。
それぞれの特徴を比較することで、どちらを選ぶべきかを考えることができます。
項目 | 街金(消費者金融) | 闇金 |
---|---|---|
運営の正当性 | 金融庁に登録された正規業者 | 無登録で違法に営業 |
金利 | 法律に基づいた上限金利を遵守 | 法定金利を超えた高金利(暴利) |
融資条件の透明性 | 融資条件が明確で、事前に説明あり | 契約内容が不透明、変更されることもある |
返済方法 | 契約に基づいた返済計画があり、返済期日も明確 | 返済計画が不明確で、強引な返済要求が行われることが多い |
安全性 | 法的保護があり、安心して利用できる | 非合法で、利用者にとって非常に危険 |
借り手の保護 | 借り手に対する保護があり、問題が発生した場合に相談できる | 借り手の権利が無視され、問題解決の手段が限られている |
4. 街金を選ぶ際の注意点
山口の激甘審査を特徴とする街金は、柔軟な審査を行っているため、審査が通りやすいというメリットがありますが、その反面、過剰にお金を借りすぎてしまわないよう注意が必要です。
特に、融資を受ける前には、返済計画をしっかりと立て、返済能力を十分に考慮した上で利用しましょう。
また、街金を選ぶ際には、金融庁に登録されている正規業者であるかを必ず確認しましょう。
無登録の業者や怪しい業者には手を出さないようにすることが、最も重要なポイントです。
山口の激甘審査の街金で審査落ち・・お金を借りれないときは
急な支払いが必要だったり、どうしても資金が足りなかったりする場合に頼りにするのが街金(消費者金融)ですが、審査に落ちてしまうこともあります。
山口の街金は審査が比較的甘いと言われていますが、それでも全ての申込みが通るわけではありません。
もし審査に落ちてしまった場合、どうすればよいのでしょうか?
1. 街金の審査基準とは?
まず、街金の審査基準について理解しておくことが大切です。
山口には数多くの街金があり、各社それぞれ異なる審査基準を設けています。
しかし、基本的な審査ポイントとしては以下のようなものがあります。
1.1 収入の安定性
最も重視されるのは「収入の安定性」です。
街金では、返済能力があるかどうかを確認するために、申込者の収入状況を詳しく調べます。
安定した仕事に就いているか、毎月一定の収入があるかどうかがポイントとなります。
1.2 クレジットカードやローンの履歴
過去にクレジットカードの支払い遅延や、他のローンが返済できなかった場合、その履歴が審査に影響を与えることがあります。
特に多重債務者や返済履歴に問題がある場合、審査に落ちる可能性が高くなります。
1.3 他社からの借り入れ状況
すでに他の金融機関や街金から借り入れをしている場合、それが返済できていないと審査に通らないことがあります。
借入総額が多すぎると、「返済能力がない」と見なされるため、審査が厳しくなることもあります。
2. 審査に落ちた理由を確認する
もし街金の審査に落ちてしまった場合、まずはその理由を確認することが重要です。
多くの場合、審査に落ちた理由は、収入が不安定、借り入れ過多、または信用情報に問題があることです。
街金の担当者に問い合わせて、具体的な理由を尋ねてみると、改善点が見えてくるかもしれません。
2.1 収入が不安定な場合
フリーターやアルバイト、契約社員などの雇用形態の場合、安定した収入が証明できないと審査に落ちることがあります。
この場合、正社員への転職や、収入の安定を図ることが大切です。
また、定期的な収入を証明するために給与明細をしっかりと準備することも重要です。
2.2 他社の借り入れが多い場合
他社からの借り入れが多すぎると、「過剰な借金を抱えている」と見なされることがあります。
これにより、街金は融資をためらうことがあります。もし多重債務に陥っている場合、返済を計画的に行い、借金を減らすことが解決への第一歩です。
2.3 信用情報に問題がある場合
信用情報に問題がある場合、審査に落ちることがあります。
例えば、過去に支払い遅延があったり、自己破産や債務整理を行った履歴があると、街金はリスクを避けるために融資を拒否することがあります。この場合、信用情報を修復するために時間が必要です。
3. 他の資金調達手段を考える
もし街金の審査に落ちた場合でも、諦める必要はありません。
他にもお金を借りる方法はありますので、代替手段を考えてみましょう。
3.1 銀行系カードローンを利用する
銀行系カードローンは、街金よりも審査が厳しい場合がありますが、その分金利が低く、長期的に返済を続けることが可能です。
特に、安定した収入があれば、街金ではなく銀行カードローンを利用することも検討してみましょう。
3.2 親や友人から借りる
親や友人に相談してお金を借りる方法もあります。
街金の審査に落ちたことを素直に伝え、借りることができるかどうかを確認してみましょう。家族や友人から借りる場合は、返済期限や金額について明確に取り決めておくことが大切です。
3.3 質屋を利用する
質屋では、物品を担保にお金を借りることができます。
例えば、宝石や高級時計、電化製品などの価値のある物品を持っている場合、それを担保にお金を借りることができます。審査が比較的緩やかで、短期間でお金を調達することが可能です。
3.4 クレジットカードのキャッシング
クレジットカードを持っている場合、そのカードのキャッシング機能を利用することができます。
これも一つの手段です。ただし、金利が高いため、借りすぎには注意が必要です。
4. 審査に通るための対策
次に、審査に通るための対策についても考えてみましょう。
審査に落ちた理由を改善することで、再度申込む際に通る可能性が高くなります。
4.1 信用情報を確認し修復する
自己破産や債務整理をしている場合、信用情報機関に記録が残っていることがあります。
もし問題がある場合は、その履歴が消えるまで待つか、問題を解決するための対策を講じることが必要です。また、自分の信用情報を確認することも一つの方法です。
4.2 借り入れ額を減らす
他社からの借り入れが多い場合、その額を減らすことが大切です。
可能であれば返済を進め、借り入れ総額を減らしてから再度申込むと良いでしょう。
4.3 収入証明をしっかりと準備する
収入が安定していることを証明するために、給与明細書や税務署からの納税証明書などをしっかりと準備しましょう。
これらをしっかりと提出することで、審査に通る可能性が高くなります。
5. 最後に
審査に落ちた場合でも、焦らずに他の資金調達手段を検討し、改善すべき点を見つけて対策を講じることが大切です。
山口の街金は審査が比較的緩やかではありますが、それでも全ての申込者が通るわけではありません。
しかし、他にも多くの選択肢があり、適切な方法を選べば問題を解決できるでしょう。